Blog
Leveraging AI to Revolutionize the Global Supply Chain Landscape
This white paper explores the diverse applications of AI in global supply chains, highlighting its potential to drive innovation, mitigate risks, and unlock new opportunities for businesses.
Details
Authors: Greg Smith Digital-SCM LLC gsmith@digital-scm.com
Larry Layden UCBOS Larry.layden@ucbos.com
The global supply chain landscape is undergoing a significant transformation, driven by the integration of Artificial Intelligence (AI) technologies. AI is revolutionizing various industries by optimizing operations, enhancing decision-making, and improving overall efficiency. This white paper explores the diverse applications of AI in global supply chains, highlighting its potential to drive innovation, mitigate risks, and unlock new opportunities for businesses. From demand forecasting and inventory management to logistics optimization, supplier relationship management, and predictive analytics, AI offers a powerful toolkit to streamline supply chain processes and deliver sustainable competitive advantages.
The integration of AI and Machine Learning (ML) technologies is reshaping how businesses manage their supply chains. AI-powered solutions offer data-driven insights and predictive capabilities, allowing organizations to optimize operations, improve accuracy, and gain a competitive edge. However, as with any transformative technology, there is a need for rigorous monitoring and validation to ensure data accuracy and reliability.
Validation of AI ML in the Supply Chain: Validation is crucial to ascertain the performance and generalizability of AI and ML models in supply chains. It helps reduce biases and potential errors, fostering transparency and trust in decision-making processes. Rigorous validation processes, such as cross-validation, stress testing, and sensitivity analysis, ensure data quality and enable users to make informed decisions while minimizing risks.
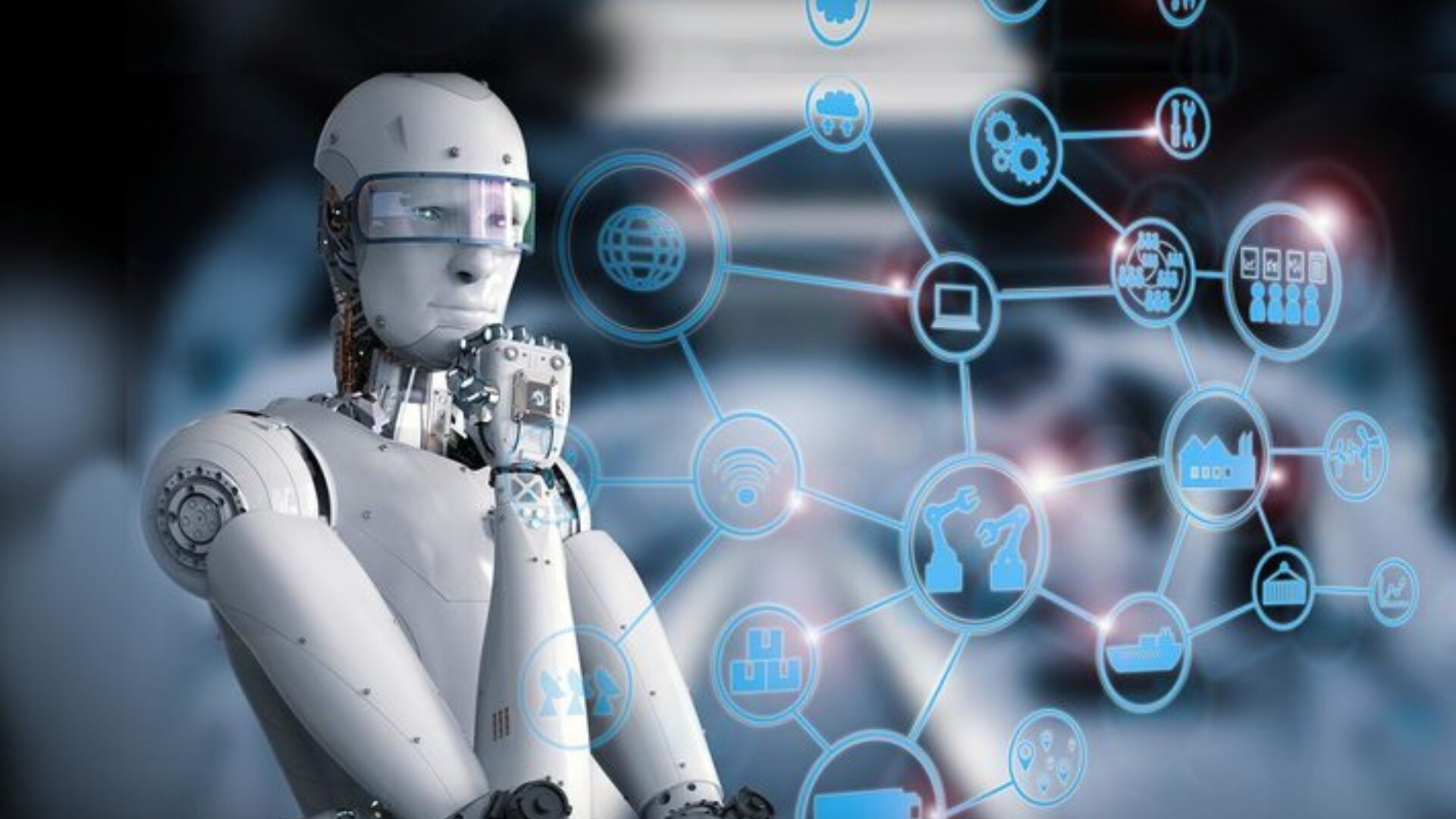
Conclusion
AI holds immense potential in transforming global supply chains, enabling businesses to achieve greater efficiency, transparency, and sustainability. To realize these benefits,embrace a data-driven and validated approach to AI implementation, ensuring accurate and reliable outcomes. As organizations continue to harness the power of AI, collaboration across industries is essential to drive innovation, resilience, and success in the dynamic global marketplace. The future of supply chains lies in leveraging AI’s potential to unlock unprecedented opportunities for growth and competitiveness.
A summary of Potential uses of AI in Supply Chan transformation and Implementation steps follow.
Potential Uses of AI in Supply Chain Transformation:
Demand Forecasting and Inventory Management: AI-driven demand forecasting provides data-driven accuracy for better decision-making, while intelligent inventory optimization improves overall efficiency in inventory management.
Predictive Analytics and Supply Chain Analytics: AI supports predictive maintenance, demand and supply planning, and supply chain risk management
Environmental, Social, and Governance (ESG) Impact: AI and ML technologies positively impact ESG functions in supply chains. These technologies enhance environmental impact through optimized operations and resource consumption, improve risk management, increase transparency and traceability, and support ethical decision-making and continuous improvement.
Logistics and Transportation Optimization: Integrating AI in route optimization and fleet management enhances efficiency, customer service, and cost savings. Real-time tracking and visibility further improve logistics operations.
Warehouse and Distribution Center Management: Warehouse automation and robotics, order fulfillment optimization, warehouse layout and space utilization, and predictive analytics in supply chain analytics are facilitated by AI.
Manufacturing: AI helps in-house and outsourced manufacturing evaluations, maintaining quality standards and compliance, and selecting outsourced manufacturing partners.
Product Lifecycle Management (PLM): AI assists data management, design and engineering optimization, collaboration, predictive analytics, quality control, supply chain integration, and automation of manual tasks.
Procurement: AI aids compliance and contract management, supplier selection, strategic decision-making, scalability, adaptability, and improved supplier relationships.
Supplier Relationship Management: AI enhances supplier selection and evaluation, risk mitigation, compliance, procurement, and contract management.
Sales and Operations Process S&OP: AI is always on automation and can remove debate over what data is right, thus creating a single version of the truth. And can provide multiple super scenarios; including achieving sustainability goals at multiple levels. Focusing seasoned stakeholders who can evaluate the smallest details, trends, or nuance and draw high-quality conclusions over their computerized counterparts.
Blockchain and AI Integration in Supply Chains: AI enhances transparency and traceability, secures supply chain data, and automates smart contracts and transactions.
Next Steps in the AI/ML Implementation Process:
Assess Current State and Define Objectives: Perform a thorough assessment of the existing supply chain processes, systems, and data sources. Understand the current pain points, inefficiencies, and areas that can benefit from AI and ML integration. Define clear objectives and specific use cases where AI and ML can add value, such as demand forecasting, inventory optimization, route optimization, or predictive maintenance.
Develop Proof of Concept (POC): Before full-scale implementation, create a POC to validate the feasibility and effectiveness of the chosen AI and ML solutions. Use historical data to run pilot tests and analyze the results. This step helps in identifying any potential challenges or adjustments needed before scaling up.
Employee Training and Change Management: Ensure that the workforce understands the benefits and limitations of these technologies and can leverage them effectively in their day-to-day operations. Provide adequate training to employees involved in using and managing AI and ML technologies.
Use a Robust Platform: Leverage the inclusive capabilities to quickly drive real results and scale easier. Implement business composable platform and dramatically eases, simplifies, and automates:
- Data Collection: Identify all the disparate supply chain nodes and data sources across the organization. Included data from inside the enterprise and external to the enterprise such as suppliers, manufacturers, warehouses, transportation, sales, telematics, and customer data.
- Data Cleaning. Preparation and Integration: Ensure the data is collected, makes sense, and associates all the data dynamically into data unified logical data model. In a timely manner. Data often comes in various formats and may contain errors or inconsistencies. Companies need data cleaning and preparation to ensure that the data is accurate, complete, and ready for analysis. This step is vital as AI and ML algorithms heavily rely on high-quality data.
- Data Security and Governance: Given the sensitive nature of supply chain data, it is essential to establish robust data security measures and governance policies. Implement access controls, encryption, and data sharing protocols to protect the data from unauthorized access or breaches.
- Select appropriate AI and ML: Use explainable and transparent capabilities to assure trust in the outcome and ease adoption.
- Build a Scalable Architecture: Architect a scalable and flexible system to accommodate the increasing volume of data and evolving business requirements. Consider a No-Code cloud-based platform quick solution, scalability and cost-effectiveness allowing the company to expand its AI and ML capabilities as needed.
- Integrate AI and ML into Supply Chain Processes: Integrate the selected AI and ML solutions into the supply chain processes seamlessly. This may involve automation of tasks, real-time decision-making capabilities, and predictive analytics to optimize the supply chain’s efficiency and responsiveness.
- Continuous Monitoring and Improvement: Implement continuous monitoring and evaluation of the AI and ML models’ performance. Regularly update and refine the algorithms to adapt to changing market dynamics and evolving supply chain requirements.
Share this article now
100% No-Code
Unlock new outcomes 10X Faster by extending your Legacy, ERP, and SCM! Don’t rip out anything!